If you have been involved in data, analytics, BI, or AI domains for at least a few years, you have undoubtedly encountered the following situation. Someone in a business unit has a need for information to inform a decision and upon starting a conversation with one of their technology colleagues is faced with a question from the latter “what data do you need?” The response often takes one of two forms, “I don’t really know; give me all the data you have” or “I need this one particular data set to address my immediate need or project.”
The response from the data professional usually addresses the question literally — delivering a data set or access to requested data via a plethora of data management and data visualization technology, from data warehouses and data lakes to dashboards and reports.
Over the years, greater processing power, more automation of data pipelines, and better data visualization have combined to continuously speed up the delivery of data to business users. One might consider that a success, but only if the speed of data delivery is the metric that matters. But does it?
What happens when data is delivered to a business user? The person may be able to look at a trendline; they will likely be able to compare the current period’s performance of any given data point to previous periods. Some organizations have software to highlight to the user anomalies or deviations from past norms; some organizations have even begun to incorporate ML-based automation to help users with autogenerated forecasts of how a given trend might evolve.
However, what this information delivery functionality does not provide is support for the process of decision making, which remains largely the responsibility of human “wetware.” We see the data and we use our experience, mental models, discrete data points from dashboards and reports to assess options that lead to a decision. This approach to the decision-making process lacks scale, governance, orchestration, and technical support for all the steps within a decision-making process — a process that does not stop with the delivery of data to an individual.
This approach to decision making is not sustainable. To achieve better decision making, it is not enough to invest in the best data lakehouse, best data science team, best AI technology, or best data visualization tools.
Our contention is that one of the biggest shortcomings plaguing most organizations is the use of the question “what data do you need?” We propose that the solution to overcoming substandard returns on investment in data, BI, and AI starts with changing the question to “what decisions do you need to make?”
For a more detailed discussion of decision intelligence challenges, opportunities and benefits see A Case for Decision Intelligence: From “What Data Is Needed?” to “What Decisions Need to Be Made?”
Decision Making
At its core, decision making is the process of orienting the decision maker, using all relevant information, toward selecting one of two or more alternatives based on probabilities of success and decision execution constraints.
- Accessing and organizing data into a form that makes data available for analysis.
- Analyzing data using descriptive and predictive methods, including AI.
- Recommending a decision: defining and presenting likely alternatives or options.
- Deciding by selecting an option based on constraints and goals.
- Executing a decision by acting based on the decision made.
- Monitoring and evaluating results of the decision and action.
Given the opportunity to accelerate decision velocity through AI-powered decision intelligence while facing technical, operational, and organizational roadblocks, leading organizations are making a clear choice in how they allocate their investments – they are tightly connecting the six steps of a decision-making process and deploying decision intelligence software.
Decision Intelligence Software
To be considered a decision intelligence software by IDC, the software product should be packaged for the purpose of fully or partially automating all steps in the decision-making process. Decision intelligence software includes capabilities for decision design, engineering, and orchestration.
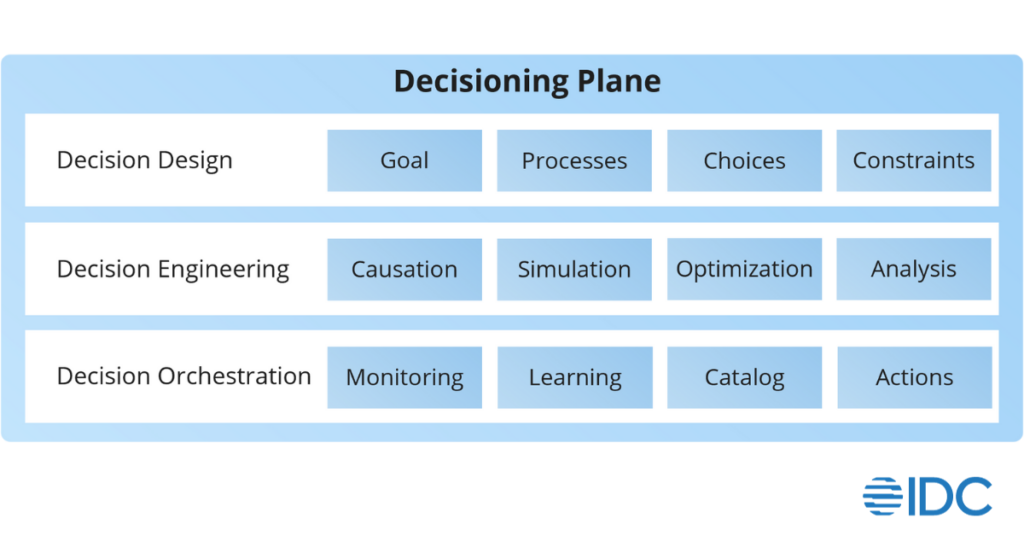
- Decision design. Decision design provides functionality for users to define business goals or desired outcomes; map decision-making workflows, including feedback loops and approval points; and identify choices and constraints that limit the options available to decision makers.
- Decision engineering. Decision engineering provides functionality to organize the available data using semantic and/or ontological frameworks, then analyze the data using a range of use case–specific methods that may include simulation, optimization, descriptive, and predictive analysis.
- Decision orchestration. Decision orchestration provides functionality to monitor all elements of the decision-making workflow (e.g., rules, algorithms, data sets, approval hierarchies, goals, constraints, decisions, and so forth) and enables their ongoing or periodic adjustment based on automated and human-generated feedback loops.
Note that to see this decisioning plane in the context of the other three planes of an enterprise intelligence architecture see IDC blog post Navigating the Planes of Enterprise Intelligence Architecture.
Together, these capabilities enable not only the collection and analysis of data, but also all the capabilities shown below.
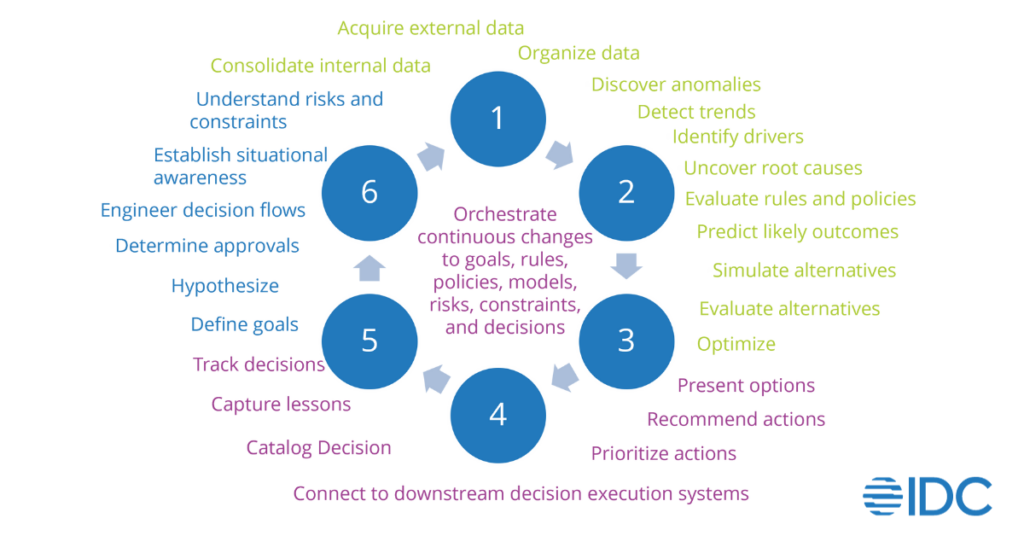
Note that IDC analysts are currently in the process of working on an assessment of a select set of decision intelligence software vendors, which will be published in Q1 2024.
Benefits and Promises: Why Should You Care About Decision Intelligence?
Every organization wants to traverse the decision-making process as fast as possible. In some cases, going through one cycle can take hours, days, weeks, or even months. In other cases, it can happen almost instantaneously. However, speed is only one factor that leads to greater decision velocity; the other is control.
We define control as decision governance, but also as quality, accuracy, and contextualization. Speed and control in decision making must be balanced. Having control of the decision-making process matters, but it cannot come at the expense of speed or the organization risks having lower adaptability when the inevitable time for course correction arrives. Decision intelligence can play a critical role in:
- Identifying decision-making processes as they exist in ongoing operations rather than as envisioned in outdated process documentation.
- Improving retention of knowledge about how decisions are made and by whom.
- Ensuring decision-making consistency across the organization and its decision makers.
Together, these benefits, in turn, are foundational to the achievement of desired business outcomes, such as increased revenue, lower costs, improved margin, and so forth. To achieve success and differentiation in the digital era, organizations should consider the following:
- Guide technologists involved in data engineering and management, and even more importantly, those involved in data analysis, data science, and AI, to pivot from asking what data their constituents need to what decisions they need to make.
- Evaluate decision attributes to identify best opportunities for decision automation and decision augmentation, respectively. Differentiate between data-driven and data-informed decision making. Highlight the role of people versus machines and shortcomings and competencies of both.
- Begin developing a cadre of staff with specialization in decision design, engineering, and orchestration.
- Invest in data literacy initiatives to ensure all staff become more comfortable with embracing uncertainty and develop a healthy skepticism of recommendations generated by opaque systems.
- Ensure the decision intelligence software provides the necessary transparency via decision monitoring and cataloging to engender trust among all users.
- Reconsider the goal of your data, analytics, and AI initiatives and investments. If they are not helping improve decision velocity, consider reallocating resources to projects that are.
- Focus on change management. One of the biggest challenges facing organizations is to address the growing gap between the exponential growth of technology for AI-powered automation of decision making and slowly evolving the ability of people to accept and adopt the new technology into their routines.