Last month my colleagues and I on the IDC data, analytics, and enterprise intelligence research team had an inquiry with a client from a large insurance company. Part of the conversation was about the unexpected increase in costs following the migration of their data warehouse to the cloud. The situation was not necessarily atypical given the many variables involved in such a migration. What surprised me was the client’s inability to answer our question about how their workloads changed on the new data warehouse from those on the old data warehouse.
It became clear that this data management professional wasn’t informed about how the data warehouse he worked on was being used. Was it primarily for BI workloads or AI workloads or both? Was it to support the client service function or the risk management function?
This situation happens more often than it should. We often talk about data silos, but rarely about internal knowledge silos about the use of data. Perhaps we should call them “silos of apathy”. There is certainly something amiss with the data culture in organizations where data management teams don’t know how data analysts or data scientists intend to use data or when the latter are unaware how their work contributes to business decision making processes.
To highlight the need for greater understanding and collaboration among data engineers, data analysts, data scientists, and all decision makers who rely on results of data analysis, we recently published an IDC study on the four planes of the enterprise intelligence architecture.
This conceptual model starts with the hypothesis that every organization wants to increase its enterprise intelligence. In the parlance of IDC, this means that every organization wants to be better than they currently are (and/or better than their competitors) in:
- Synthesizing information
- Collectively learning
- Delivering insights at scale
- Fostering a data culture
Our hundreds of interviews with decision makers across industries and analysis of responses from thousands of survey participants across the world have identified these four capabilities as core pillars that define enterprise intelligence. These capabilities are also measurable and help differentiate organizations that are better able to leverage data, analytics, and AI to achieve their goals.
Yet, many organizations continue to address their internal demand for data-driven decision making with discrete projects optimized for KPIs that are disconnected from the goal of lifting enterprise intelligence. These organizations build large data lakehouses, invest in the best data scientists and machine learning tools, experiment with the latest generative AI, conduct data literacy training, deploying intuitive dashboards, and implement data governance policies. What they don’t do enough is connect the dots – among different technologies, different decision-making processes, different plans, data or model ops initiatives.
Our research shows that few organizations have a comprehensive view that enables execution of the enterprise intelligence strategy with the corresponding architecture that can truly improve metrics that matter. This matters because of the growing complexity across data, analytics, AI, and decision-making vectors has resulted in organizations having issues that were highlighted in IDC’s recent Data Valuation study, where respondents cited:
- Data decay: 75% of decision makers say that data loses its value within days.
- Data waste: 33% of executives say they often don’t get around to using data they receive.
- Data disconnect: 61% of executives say data complexity has increased compared to last year.
An enterprise intelligence strategy defines a corresponding architecture that becomes a guide to greater utilization of data for productive purposes, including greater decision velocity that drives differentiation in the digital era. IDC’s Future of Enterprise Intelligence research has found that organizations with greater intelligence have 3x-4x better business outcomes than their counterparts with nascent enterprise intelligence.
The IDC enterprise intelligence architecture is a conceptual representation of attributes, technologies, and functionality that enable the organization to execute its enterprise intelligence strategy. Our work on this view of the enterprise intelligence architecture began by defining the data control plane and evolved into four planes.
IDC Enterprise Intelligence Conceptual Architecture
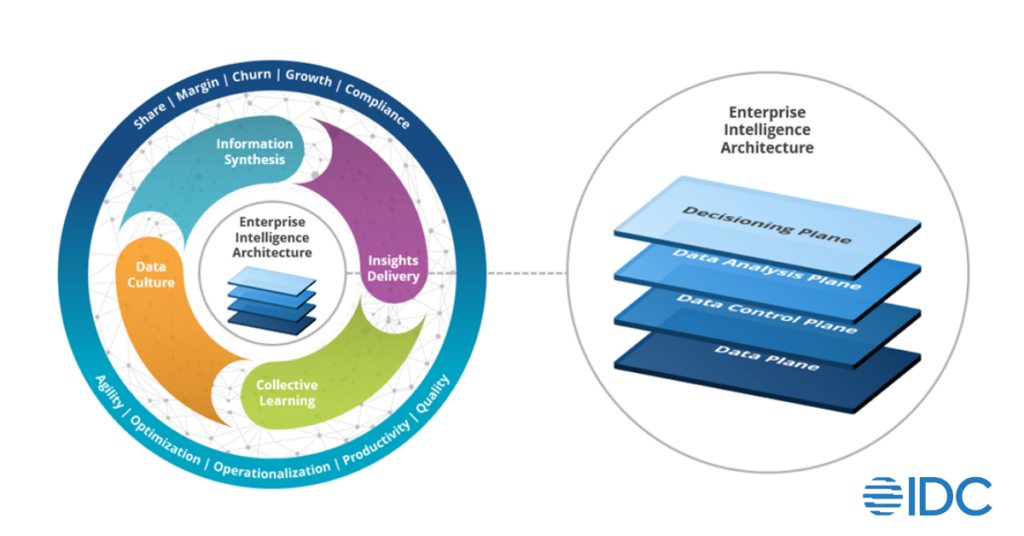
- Data Plane: Organizes the realities of modern data environments into three primary categories: distributed, diverse, and dynamic data. DBAs and data architects are the personas usually associated with the data plane.
- Data Control Plane: Leverages intelligence about data to take control of modern data environments through governance and engineering. Data engineers, data stewards, and data ops managers are typically involved in this plane.
- Data Analysis Plane: Helps organizations explore, explain, and envision data and insights. Data scientists and data analysts, BI developers, and business analysts work in the data analysis plane.
- Decisioning Plane: Has capabilities that enable decision design, engineering, and orchestration. This plane is the broadest in its use by business decision makers, executives, and even automated decisioning-systems.
Attributes, technologies, and functionality of each plane are described in greater detail in Four Planes of Enterprise Intelligence Architecture: A Conceptual View into the Data Plane, Data Control Plane, Data Analysis Plane, and Decisioning Plane, where they are depicted along with commons services across the planes (e.g. security, monitoring, knowledge management, etc.)
Attributes of the Four Planes of the Enterprise Intelligence Architecture
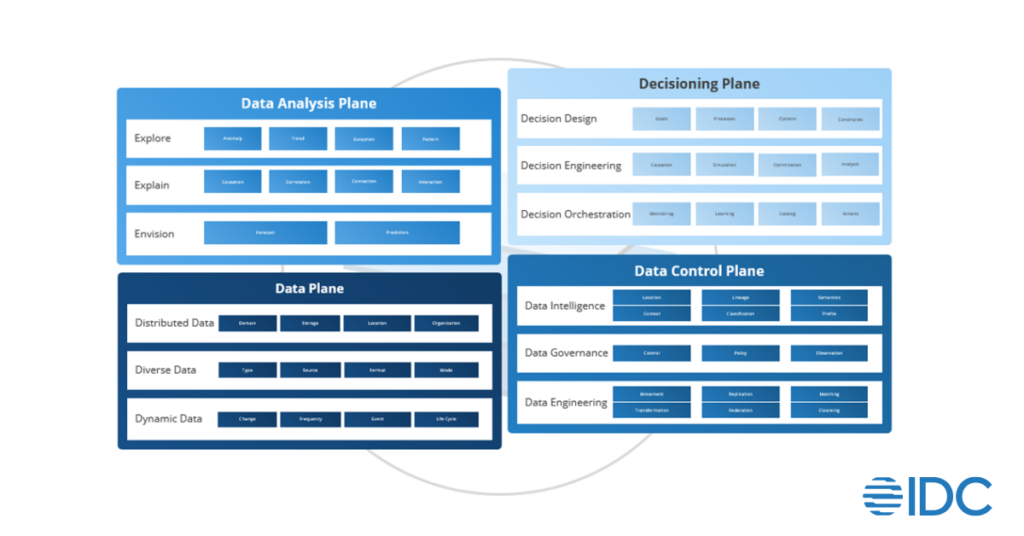
The four planes are also aligned with personas who must collaborate to achieve common goals rather than only optimize for peak performance within their plane.
Very few vendors address all the planes fully with packaged software, thus one of the considerations in evaluating technology providers and their products is to understand how the vendor moves or evolves within and across planes. Some vendors expand their portfolios and functionality through internal R&D, others do so through acquisitions. Extra caution is warranted when a vendor ‘skips’ a plane. For example, when they have been providing technology for the data plane and expand into the data analysis or decisioning planes. These types of moves are difficult and rarely successful.
When vendors don’t fully address a plane, customers must substitute a product from another vendor, or develop their own technology – often based on an open-source project, and often focused on immediate needs rather that strategic direction of the enterprise intelligence architecture. Whether integration of multiple software components is intentional or involuntary, it creates overhead and risks due to integration and ongoing maintenance needs. To counter such risks, it’s important to understand the extent to which a vendor’s product provides support of open data and analysis standards.
Focus your organization’s enterprise intelligence strategy to be top-down from the decisioning plane to the data plane. Too many organizations do the opposite and end up with projects resulting in great data management technology solutions that are disconnected from the goal of improving overall enterprise intelligence.