Hyper-experimentation with Generative AI (GenAI) dominated the conversations of business and technology leaders in organizations of all sizes, across all industries, and in all countries for the past 18 months.
Checking in with CIOs and business leaders 18 months later, we can report that a typical enterprise identified hundreds of GenAI use cases. They launched dozens of Proofs of Concept (POCs), but they put less than six into production, so far. This GenAI scramble is not sustainable for enterprises, or for technology providers who care about converting POCs into sustainable, long-term business.
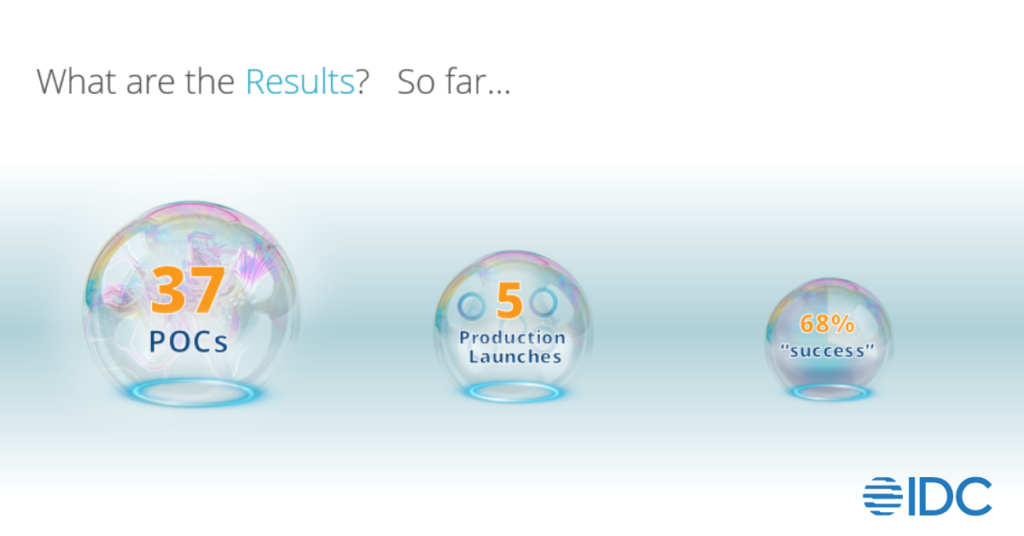
Is it time to write off GenAI as just another over-hyped tech story that generates lots of bubbles, but doesn’t have a lasting impact? Uh…no. Doing so would also be a big mistake.
The focus on GenAI experimentation obscures the reality that most organizations are already invested in AI across their business. We surveyed 889 IT leaders in May 2024, and 84% believed (42% strongly) that AI/GenAI is the next strategic corporate workload like ERP or ecommerce was before. AI is already embedded into how they engage with customers, how they monitor activities in their factories and warehouses, and how they automate tasks such as “procure to pay”. They also know that securing employees’ devices and their critical systems depends upon aggressive use of AI by security product and services providers.
The casting of a wide net when it comes to GenAI experimentation increases your CIOs’ awareness of the extent of overall AI use, but also shows how fragmented and even duplicative that use is. Your business and IT leaders setting 2025 tech investment plans need to develop an enterprise wide-AI strategy, building on the “lessons learned” while doubling down on the demonstrated benefits of GenAI for boosting business outcomes.
2025 will be the year of the AI Pivot. How effectively you set priorities, make decisions, and address barriers will decide if you are ready to fuel business growth on an AI foundation or will still be racing to catch up a couple years from now.
Where should you start? IDC’s AI Adoption Model
How severely were your GenAI experimentation efforts limited in the following areas?
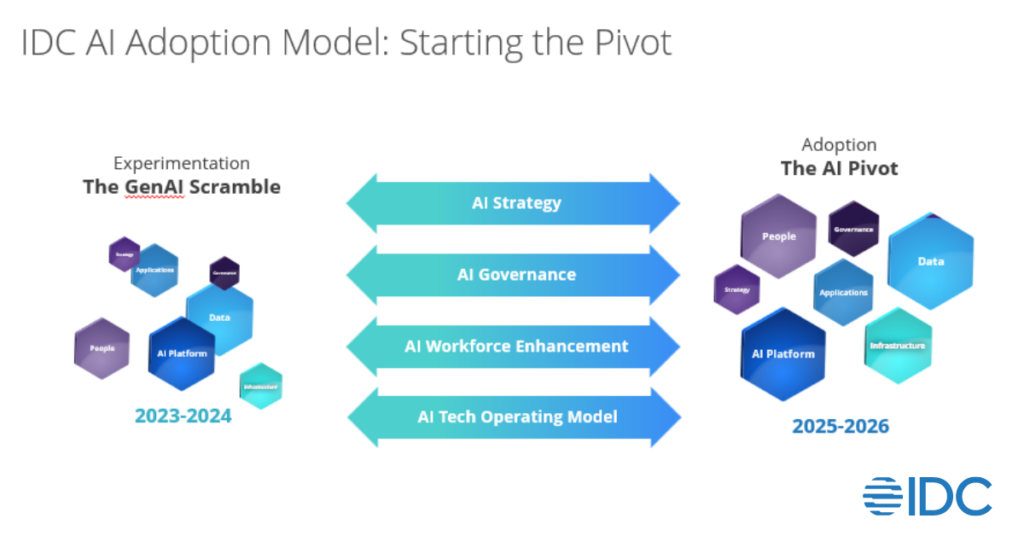
Strategy: Depth of relationship between business and tech teams in PoC prioritization, development, and execution is a key success factor. Poor coordination between IT and lines of business (LOBs) is one of the most often cited factors contributing to low success rates. My colleague Ewa Zborowska has some good suggestions on how to reduce Pilotitis. The critical next step? Building a use case prioritization roadmap.
Governance: GenAI experimentation across functions and with ties to multiple data sets overwhelms narrow, siloed IT governance processes. Organizations with high success rates noted their ability to quickly integrate responsible AI into strong, comprehensive governance practices. Especially important, they have solid, cross-functional data-sharing governance practices.
People: Most early POCs focus on individual actions or basic process improvements, exposing significant if under-appreciated bottlenecks. What is most exposed, however? Disconnects between senior executives and employees on the consequences. IT teams are caught in the middle as promised productivity gains fall short due to lack of training or fear of consequences.
Apps: “Now (or Coming Soon) with GenAI” is a recurring theme for technology providers. The infusion of GenAI into business, IT Ops, data management, and developer apps affects decisions on which POCs to pursue and raises the stakes in “build versus buy” decisions for production launches. The biggest ask? “Please, help us show quantifiable value!”
AI Platforms: Companies are already using diverse AI platform components across a wide range of individual AI efforts. The GenAI scramble increases the use of disparate and nascent tools and technologies across AI and GenAI specific lifecycles strains resources. What’s missing? Reusability and scale.
Data: The GenAI scramble highlights the importance and exposes weakness when it comes to identifying, quality assuring, and integrating data sets for production launches. Access to high quality data contributes to high rates of success. Past decisions to treat data during app development as a byproduct or waste product, with no thought about the importance of metadata, however, means too much “dark data”.
Infrastructure: Despite the hype about lack of access to infrastructure (extremely expensive GPUs), most enterprises do not see this as a major issue. Currently, siloed infrastructure solutions and existing as-a-Service funding models support hyper-experimentation. Where they fall short is scaling for production. Excessive costs “at scale” break ROI calculations.
What’s Next?
Prioritizing goals and investments will vary depending upon how significantly you were affected in all 7 areas. The AI pivot is about reaching the end states you need to succeed in each.
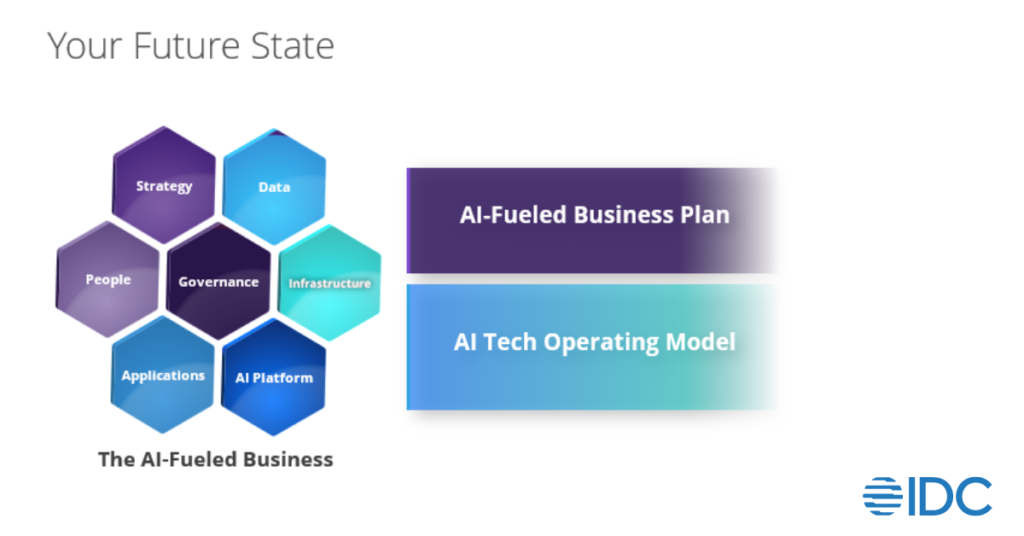
You are ready to accelerate business growth and competitive success with an AI-fueled business operating plan covering organization, culture, resources and operations. AI, not just GenAI, is fully integrated into your enterprise business strategy. It includes a targeted set of AI/GenAI super use cases that deliver maximum business impact across multiple processes and domains. You are also setting up a multi-stakeholder, unified AI governance model that aligns with your AI-fueled strategy. Most importantly, you are ensuring that effective use of AI assistants, advisors, and agents is at the core of AI-aware workforce planning and training.
Of course, setting up an AI-fueled business model is irrelevant if you aren’t shifting to an AI-ready technology operating model, ensuring that you can cost effectively and securely scale the use of AI capabilities anywhere. You are confident that you can track the costs and benefits associated with AI-infused processes & apps. You are moving towards adoption of a unified AI platform that improves data and model use as well as app dev/deployment.
You are addressing the “dark data” with AI-Ready Data based on the adoption of a “managing data as a product” strategy, ensuring that quality, accessibility, and governance of data isn’t an afterthought. Finally, infrastructure is no longer siloed, and scaling costs are no longer an impossibly high barrier to innovation. Your tech operating model is built on infrastructure that is interoperable, fit-for-purpose, and intelligently optimizable based on workload-specific cost, placement, and scale requirements.
Executing an AI Pivot may appear daunting and will require commitment across the organization, but there is good news.
- Hyper-experimentation was a necessary step. You have more clarity about GenAI uses in your organization, and you gained that clarity much faster than companies ever have before.
- GenAI tools and techniques advanced significantly during hyper-experimentation, making it faster, cheaper and easier to roll out prioritized super uses cases.
- You’ve gained more experience with the potential benefits and disruptions of GenAI in your own POCs and in the products/services you are using from tech suppliers.
IDC is ready to help you quickly assimilate all the “lessons learned” and help you find the products, services, and partners that can help you execute the AI pivot and accelerate to the next level.
Discover how IDC’s AI Use Case Discovery Tool can elevate your AI strategy—learn more here.