Chandana Gopal, IDC’s Future of Intelligence Agenda Program Lead, contributed to this blog.
“We have more than 150 applications that were written in different platforms,” said a senior director of a biotech company in a recent discussion with IDC analysts. “This makes sharing data across the enterprise difficult; understanding where the data lives, what its purpose is, what it looks like, and how to sort it, are all difficult.” In describing their initiative to overcome these and other data difficulties, this senior director talked about the discovery of a lot of obsolete data, which contributed to the challenges of separating signals from noise and ensuring that the right data is used to drive enterprise intelligence.
A vast majority of executives who are investing in intelligence want to be more agile than their competitors, have intelligence that allows everyone in the organization to ideate and innovate, and allow the organization to learn from the past and accelerate toward the future. In our conversations with senior executives, a theme that emerges time and again is that having control over data is fundamental for any organization wanting to compete in today’s digital economy.
Data has often been compared to water, and like water, data is a resource without which we, both enterprises and individuals, can’t survive or thrive. We need data to drive our actions. We need data to raise the level of enterprise intelligence. And, we need data to improve everyone’s data literacy. But, we can also drown in it and lose control of data.
In a recent call with a manufacturing company, their VP of IT in charge of data started the conversation by saying “we have a data puddle problem.” As I and my fellow market analysts at IDC thought about the comment, we realized that puddles are a great analogy for the realities of modern data environments. Have you ever found puddles to be evenly distributed, or found two puddles with the exact same circumference, depth, and water clarity? Puddles aren’t usually connected to each other, but perhaps were, before they got smaller due to evaporation… into clouds. Ever found one cloud that looks exactly the same as the other… we could go on and on with this analogy.
Making sense of all the data is difficult in a modern data environment, where data is highly distributed, diverse and dynamic. How else can we explain the results of a recent IDC research into enterprise data culture, in which half the people said they are overwhelmed by the amount of data, while at the same time 44% say they don’t have enough data to support decision making? The situation is made more difficult by demands from a new generation of data-native workers distributed throughout the enterprise that use data irrespective of their roles or level of formal training.
A more distributed, diverse, and dynamic workforce using distributed, diverse, and dynamic data has led to distributed, diverse, and dynamic data engineering, intelligence, and protection solutions. This has resulted in complex data environments and a need to have greater control over them. As one Chief Data Officer said in a recent conversation with our team, “I was brought in to provide a new level of discipline for our data estate and enterprise intelligence capabilities.”
The one certainty is that the complexity is only going to increase over time and data professionals need strategies and a common language to describe and devise a solution to it.
Terms such as data platform, data fabric, and data mesh have emerged to describe the portfolios of tools that deliver capabilities that are intended to solve modern data environment challenges. However, the term platform is overused and ambiguous, and although fabric and/or mesh help describe the interwoven characteristics of modern data environments, these terms only speak to the data and do not address the functionality of data engineering, intelligence and protection. The Data Asset Management Association (DAMA.org) defines four types of data management activities: planning, control, development, and operations. The activity that aligns best with many of the capabilities delivered by data integration and intelligence software vendors is control to manage data in a distributed plane. At IDC, we define the technology to carry out all four of these activities as a data control plane.
The data control plane is an architectural layer that sits over an end-to-end set of data activities (e.g., integration, access, governance, and protection) to manage and control the holistic behavior of people and processes in the use of distributed, diverse, and dynamic data. The term data control plane adds the missing functional characteristics of a fabric or mesh, and it accounts for the notion of distributed data across a plane of hybrid cloud environments. It has three layers as shown in this figure.
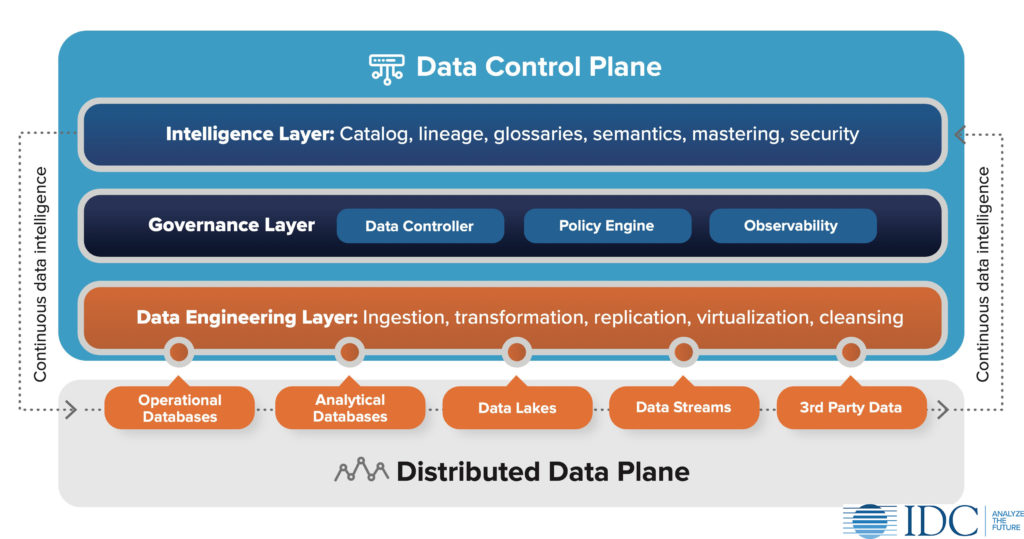
The intelligence layer leverages business, technical, relational, and operational metadata to provide transparency of data profiles, classification, quality, location, lineage, and context — thus enabling people, processes, and technology with trustworthy and reliable data. Intelligence about data, data engineering, and data usage is harvested across the distributed data plane and channels of consumption.
The governance layer of the data control plane is a conduit for control and monitoring of data intelligence and engineering activities. A data controller leverages the data intelligence layer and policy engine to control data accessibility, movement, usability, and protection. A policy engine contains, at a minimum, the rules of engagement for data, and acceptable tolerances for data drift and shift. Observability refers to continuously monitoring and testing data to determine how and when data is drifting and shifting within pipelines.
Capabilities in the data engineering layer have historically been associated with data integration, but we are seeing slightly different movement and terms being used in modern data environments. Ingestion and transformation are replacing ETL, in-memory data virtualization is federating data, and replication is one of many sources of streaming data.
The distributed data plane includes application and transactional databases, analytical databases including data warehouses, data lakes, streaming data pipelines, and data procured from third parties, either through replication or real-time access via API.
If your organization is competing in the digital economy, you may have data streams, lakes, or warehouses, and likely a lot of data puddles. Many users, and indeed administrators, of data tend to think of the various elements of data and intelligence in discrete terms and may fail to see the relationships among all the architectural components. Adopting a data control plane can yield clear business benefits, including better data quality, availability, and security, and ultimate elevate enterprise intelligence.
More detail about the data control plane architecture and related market research that looks at the current level of DataOps adoption is available on IDC.com. Stay tuned to IDC as we continue to develop deeper insights, including best practices, in raising levels of enterprise intelligence and the enabling data, analytics, machine learning, and decisioning capabilities.
Learn more about IDC’s Future of Intelligence practice, and the rest of our CEO agenda: